Cross Industry Standard Process for Data Mining
Cross Industry Standard Process for Data Mining, commonly known by its acronym CRISP-DM,[1] was a data mining process model that describes commonly used approaches that data mining experts use to tackle problems. Polls conducted at one and the same website (KDNuggets) in 2002, 2004, 2007 and 2014 show that it was the leading methodology used by industry data miners who decided to respond to the survey.[2][3][4][5] The only other data mining standard named in these polls was SEMMA. However, 3-4 times as many people reported using CRISP-DM. A review and critique of data mining process models in 2009 called the CRISP-DM the "de facto standard for developing data mining and knowledge discovery projects."[6] Other reviews of CRISP-DM and data mining process models include Kurgan and Musilek's 2006 review,[7] and Azevedo and Santos' 2008 comparison of CRISP-DM and SEMMA.[8] Efforts to update the methodology started in 2006, but have As of 30 June 2015 not led to a new version, and the "Special Interest Group" (SIG) responsible along with the website has long disappeared (see History of CRISP-DM).
In 2015, IBM Corporation released a new methodology called Analytics Solutions Unified Method for Data Mining/Predictive Analytics (also known as ASUM-DM) which refines and extends CRISP-DM.
Major phases
CRISP-DM breaks the process of data mining into six major phases.[9]
The sequence of the phases is not strict and moving back and forth between different phases is always required. The arrows in the process diagram indicate the most important and frequent dependencies between phases. The outer circle in the diagram symbolizes the cyclic nature of data mining itself. A data mining process continues after a solution has been deployed. The lessons learned during the process can trigger new, often more focused business questions and subsequent data mining processes will benefit from the experiences of previous ones.
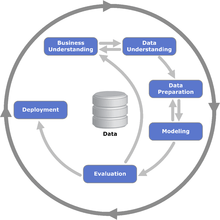
- Business Understanding
- This initial phase focuses on understanding the project objectives and requirements from a business perspective, and then converting this knowledge into a data mining problem definition, and a preliminary plan designed to achieve the objectives. A decision model, especially one built using the Decision Model and Notation standard can be used.
- Data Understanding
- The data understanding phase starts with an initial data collection and proceeds with activities in order to get familiar with the data, to identify data quality problems, to discover first insights into the data, or to detect interesting subsets to form hypotheses for hidden information.
- Data Preparation
- The data preparation phase covers all activities to construct the final dataset (data that will be fed into the modeling tool(s)) from the initial raw data. Data preparation tasks are likely to be performed multiple times, and not in any prescribed order. Tasks include table, record, and attribute selection as well as transformation and cleaning of data for modeling tools.
- Modeling
- In this phase, various modeling techniques are selected and applied, and their parameters are calibrated to optimal values. Typically, there are several techniques for the same data mining problem type. Some techniques have specific requirements on the form of data. Therefore, stepping back to the data preparation phase is often needed.
- Evaluation
- At this stage in the project you have built a model (or models) that appears to have high quality, from a data analysis perspective. Before proceeding to final deployment of the model, it is important to more thoroughly evaluate the model, and review the steps executed to construct the model, to be certain it properly achieves the business objectives. A key objective is to determine if there is some important business issue that has not been sufficiently considered. At the end of this phase, a decision on the use of the data mining results should be reached.
- Deployment
- Creation of the model is generally not the end of the project. Even if the purpose of the model is to increase knowledge of the data, the knowledge gained will need to be organized and presented in a way that is useful to the customer. Depending on the requirements, the deployment phase can be as simple as generating a report or as complex as implementing a repeatable data scoring (e.g. segment allocation) or data mining process. In many cases it will be the customer, not the data analyst, who will carry out the deployment steps. Even if the analyst deploys the model it is important for the customer to understand up front the actions which will need to be carried out in order to actually make use of the created models.
History
CRISP-DM was conceived in 1996. In 1997 it got underway as a European Union project under the ESPRIT funding initiative. The project was led by five companies: SPSS, Teradata, Daimler AG, NCR Corporation and OHRA, an insurance company.
This core consortium brought different experiences to the project: ISL, later acquired and merged into SPSS Inc. The computer giant NCR Corporation produced the Teradata data warehouse and its own data mining software. Daimler-Benz had a significant data mining team. OHRA was just starting to explore the potential use of data mining.
The first version of the methodology was presented at the 4th CRISP-DM SIG Workshop in Brussels in March 1999,[10] and published as a step-by-step data mining guide later that year.[11]
Between 2006 and 2008 a CRISP-DM 2.0 SIG was formed and there were discussions about updating the CRISP-DM process model.[6][12] The current status of these efforts is not known. However, the original crisp-dm.org website cited in the reviews,[7][8] and the CRISP-DM 2.0 SIG website[6][12] are both no longer active.
While many non-IBM data mining practitioners use CRISP-DM,[2][3][4][6] IBM is the primary corporation that currently embraces the CRISP-DM process model. It makes some of the old CRISP-DM documents available for download[11] and it has incorporated it into its SPSS Modeler product.
References
- ↑ Shearer C., The CRISP-DM model: the new blueprint for data mining, J Data Warehousing (2000); 5:13—22.
- 1 2 Gregory Piatetsky-Shapiro (2002); KDnuggets Methodology Poll
- 1 2 Gregory Piatetsky-Shapiro (2004); KDnuggets Methodology Poll
- 1 2 Gregory Piatetsky-Shapiro (2007); KDnuggets Methodology Poll
- ↑ Gregory Piatetsky-Shapiro (2014); KDnuggets Methodology Poll
- 1 2 3 4 Óscar Marbán, Gonzalo Mariscal and Javier Segovia (2009); A Data Mining & Knowledge Discovery Process Model. In Data Mining and Knowledge Discovery in Real Life Applications, Book edited by: Julio Ponce and Adem Karahoca, ISBN 978-3-902613-53-0, pp. 438-453, February 2009, I-Tech, Vienna, Austria.
- 1 2 Lukasz Kurgan and Petr Musilek (2006); A survey of Knowledge Discovery and Data Mining process models. The Knowledge Engineering Review. Volume 21 Issue 1, March 2006, pp 1 - 24, Cambridge University Press, New York, NY, USA doi: 10.1017/S0269888906000737.
- 1 2 Azevedo, A. and Santos, M. F. (2008);KDD, SEMMA and CRISP-DM: a parallel overview. In Proceedings of the IADIS European Conference on Data Mining 2008, pp 182-185.
- ↑ Harper, Gavin; Stephen D. Pickett (August 2006). "Methods for mining HTS data". Drug Discovery Today. 11 (15–16): 694–699. doi:10.1016/j.drudis.2006.06.006. PMID 16846796.
- ↑ Pete Chapman (1999); The CRISP-DM User Guide.
- 1 2 Pete Chapman, Julian Clinton, Randy Kerber, Thomas Khabaza, Thomas Reinartz, Colin Shearer, and Rüdiger Wirth (2000); CRISP-DM 1.0 Step-by-step data mining guides.
- 1 2 Colin Shearer (2006); First CRISP-DM 2.0 Workshop Held