Convolutional neural network
Machine learning and data mining |
---|
![]() |
Machine learning venues
|
|
In machine learning, a convolutional neural network (CNN, or ConvNet) is a type of feed-forward artificial neural network in which the connectivity pattern between its neurons is inspired by the organization of the animal visual cortex. Individual cortical neurons respond to stimuli in a restricted region of space known as the receptive field. The receptive fields of different neurons partially overlap such that they tile the visual field. The response of an individual neuron to stimuli within its receptive field can be approximated mathematically by a convolution operation.[1] Convolutional networks were inspired by biological processes[2] and are variations of multilayer perceptrons designed to use minimal amounts of preprocessing.[3] They have wide applications in image and video recognition, recommender systems[4] and natural language processing.[5]
The convolutional neural network is also known as shift invariant or space invariant artificial neural network (SIANN), which is named based on its shared weights architecture and translation invariance characteristics.[6][7]
Overview
Convolutional neural networks model animal visual perception, and can be applied to visual recognition tasks.
Image recognition
Convolutional neural networks (CNNs) consist of multiple layers of receptive fields. These are small neuron collections which process portions of the input image. The outputs of these collections are then tiled so that their input regions overlap, to obtain a better representation of the original image; this is repeated for every such layer. Tiling allows CNNs to tolerate translation of the input image.[8]
Convolutional networks may include local or global pooling layers, which combine the outputs of neuron clusters.[9][10] They also consist of various combinations of convolutional and fully connected layers, with pointwise nonlinearity applied at the end of or after each layer.[11] A convolution operation on small regions of input is introduced to reduce the number of free parameters and improve generalization. One major advantage of convolutional networks is the use of shared weight in convolutional layers, which means that the same filter (weights bank) is used for each pixel in the layer; this both reduces memory footprint and improves performance.[3]
Time delay neural networks
Some time delay neural networks also use a very similar architecture to convolutional neural networks, especially those for image recognition or classification tasks, since the tiling of neuron outputs can be done in timed stages, in a manner useful for analysis of images.[12]
Compared to other image classification algorithms, convolutional neural networks use relatively little pre-processing. This means that the network is responsible for learning the filters that in traditional algorithms were hand-engineered. The lack of dependence on prior knowledge and human effort in designing features is a major advantage for CNNs.
History
The design of convolutional neural networks follows visual mechanisms in living organisms.
Receptive fields
Work by Hubel and Wiesel in the 1950s and 1960s showed that cat and monkey visual cortex contains neurons that individually respond to small regions of the visual field. Provided the eyes are not moving, the region of visual space within which visual stimuli affect the firing of a single neuron is known as its receptive fields. Neighboring cells have similar and overlapping receptive field. Receptive field size and location varies systematically across the cortex to form a complete map of visual space, the cortex in each hemisphere representing the contralateral visual field.
Their 1968 paper[13] identified two basic visual cell types in the brain:
- simple cells, whose output is maximized by straight edges having particular orientations within their receptive field
- complex cells, which have larger receptive fields, whose output is insensitive to the exact position of the edges in the field.
Neocognitron
The neocognitron [14] was introduced in 1980.[11][15] The neocognitron does not require units located at several network positions to have the same trainable weights. This idea appears in 1986 in the book version of the original backpropagation paper[16] (Figure 14). They were developed in 1988 for temporal signals.[17] Their design was improved in 1998,[18] generalized in 2003,[19] and simplified in the same year.[20]
LeNet-5
LeNet-5, a pioneering 7-level convolutional network by Yann LeCun et al.[18] that classifies digits, is applied by several banks to recognise hand-written numbers on checks (cheques) digitized in 32x32 pixel images. The ability to process higher resolution images requires larger and more convolutional layers, so this technique is constrained by the limited availability of computing resources.
Shift-invariant neural network
Similarly, a shift invariant neural network was proposed for image character recognition in 1988.[6][7] The architecture and training algorithm were modified in 1991[21] and applied for medical image processing[22] and automatic detection of breast cancer in mammograms.[23]
A different convolution-based design was proposed in 1988[24] for application to decomposition of one-dimensional electromyography convolved signals via de-convolution. This design was modified in 1989 to other de-convolution-based designs.[25][26]
Following the 2005 paper that established the value of GPGPU for machine learning,[27] several publications described more efficient ways to train convolutional neural networks using GPU computing.[28][29][30][31] In 2011, they were refined and implemented on a GPU, with impressive results.[9] In 2012, Ciresan et al. significantly improved on the best performance in the literature for multiple image databases, including the MNIST database, the NORB database, the HWDB1.0 dataset (Chinese characters), the CIFAR10 dataset (dataset of 60000 32x32 labeled RGB images),[11] and the ImageNet dataset.[32]
Distinguishing features
While traditional multilayer perceptron (MLP) models were successfully used for image recognition, due to the full connectivity between nodes they suffer from the curse of dimensionality and thus do not scale well to higher resolution images.
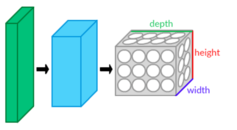
For example, in CIFAR-10, images are only of size 32x32x3 (32 wide, 32 high, 3 color channels), so a single fully connected neuron in a first hidden layer of a regular neural network would have 32*32*3 = 3,072 weights. A 200x200 image, however, would lead to neurons that have 200*200*3 = 120,000 weights.
Such network architecture does not take into account the spatial structure of data, treating input pixels which are far apart and close together on exactly the same footing. Clearly, the full connectivity of neurons is wasteful in the framework of image recognition, and the huge number of parameters quickly leads to overfitting.
Convolutional neural networks are biologically inspired variants of multilayer perceptrons, designed to emulate the behaviour of a visual cortex. These models mitigate the challenges posed by the MLP architecture by exploiting the strong spatially local correlation present in natural images. As opposed to MLPs, CNN have the following distinguishing features:
- 3D volumes of neurons. The layers of a CNN have neurons arranged in 3 dimensions: width, height and depth. The neurons inside a layer are only connected to a small region of the layer before it, called a receptive field. Distinct types of layers, both locally and completely connected, are stacked to form a CNN architecture.
- Local connectivity: following the concept of receptive fields, CNNs exploit spatially local correlation by enforcing a local connectivity pattern between neurons of adjacent layers. The architecture thus ensures that the learnt "filters" produce the strongest response to a spatially local input pattern. Stacking many such layers leads to non-linear "filters" that become increasingly "global" (i.e. responsive to a larger region of pixel space). This allows the network to first create good representations of small parts of the input, then assemble representations of larger areas from them.
- Shared weights: In CNNs, each filter is replicated across the entire visual field. These replicated units share the same parameterization (weight vector and bias) and form a feature map. This means that all the neurons in a given convolutional layer detect exactly the same feature. Replicating units in this way allows for features to be detected regardless of their position in the visual field, thus constituting the property of translation invariance.
Together, these properties allow convolutional neural networks to achieve better generalization on vision problems. Weight sharing also helps by dramatically reducing the number of free parameters being learnt, thus lowering the memory requirements for running the network. Decreasing the memory footprint allows the training of larger, more powerful networks.
Building blocks
A CNN architecture is formed by a stack of distinct layers that transform the input volume into an output volume (e.g. holding the class scores) through a differentiable function. A few distinct types of layers are commonly used. We discuss them further below:
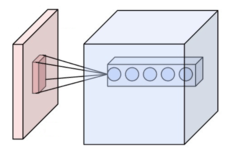
Convolutional layer
The convolutional layer is the core building block of a CNN. The layer's parameters consist of a set of learnable filters (or kernels), which have a small receptive field, but extend through the full depth of the input volume. During the forward pass, each filter is convolved across the width and height of the input volume, computing the dot product between the entries of the filter and the input and producing a 2-dimensional activation map of that filter. As a result, the network learns filters that activate when it detects some specific type of feature at some spatial position in the input.
Stacking the activation maps for all filters along the depth dimension forms the full output volume of the convolution layer. Every entry in the output volume can thus also be interpreted as an output of a neuron that looks at a small region in the input and shares parameters with neurons in the same activation map.
Local connectivity
When dealing with high-dimensional inputs such as images, it is impractical to connect neurons to all neurons in the previous volume because such a network architecture does not take the spatial structure of the data into account. Convolutional networks exploit spatially local correlation by enforcing a local connectivity pattern between neurons of adjacent layers: each neuron is connected to only a small region of the input volume. The extent of this connectivity is a hyperparameter called the receptive field of the neuron. The connections are local in space (along width and height), but always extend along the entire depth of the input volume. Such an architecture ensures that the learnt filters produce the strongest response to a spatially local input pattern.
Spatial arrangement
Three hyperparameters control the size of the output volume of the convolutional layer: the depth, stride and zero-padding.
- Depth of the output volume controls the number of neurons in the layer that connect to the same region of the input volume. All of these neurons will learn to activate for different features in the input. For example, if the first Convolutional Layer takes the raw image as input, then different neurons along the depth dimension may activate in the presence of various oriented edges, or blobs of color.
- Stride controls how depth columns around the spatial dimensions (width and height) are allocated. When the stride is 1, a new depth column of neurons is allocated to spatial positions only 1 spatial unit apart. This leads to heavily overlapping receptive fields between the columns, and also to large output volumes. Conversely, if higher strides are used then the receptive fields will overlap less and the resulting output volume will have smaller dimensions spatially.
- Sometimes it is convenient to pad the input with zeros on the border of the input volume. The size of this zero-padding is a third hyperparameter. Zero padding provides control of the output volume spatial size. In particular, sometimes it is desirable to exactly preserve the spatial size of the input volume.
The spatial size of the output volume can be computed as a function of the input volume size , the kernel field size of the Conv Layer neurons , the stride with which they are applied , and the amount of zero padding used on the border. The formula for calculating how many neurons "fit" in a given volume is given by . If this number is not an integer, then the strides are set incorrectly and the neurons cannot be tiled to fit across the input volume in a symmetric way. In general, setting zero padding to be when the stride is ensures that the input volume and output volume will have the same size spatially. Though it's generally not completely necessary to use up all of the neurons of the previous layer, for example, you may decide to use just a portion of padding.
Parameter sharing
Parameter sharing scheme is used in convolutional layers to control the number of free parameters. It relies on one reasonable assumption: That if one patch feature is useful to compute at some spatial position, then it should also be useful to compute at a different position. In other words, denoting a single 2-dimensional slice of depth as a depth slice, we constrain the neurons in each depth slice to use the same weights and bias.
Since all neurons in a single depth slice are sharing the same parametrization, then the forward pass in each depth slice of the CONV layer can be computed as a convolution of the neuron's weights with the input volume (hence the name: convolutional layer). Therefore, it is common to refer to the sets of weights as a filter (or a kernel), which is convolved with the input. The result of this convolution is an activation map, and the set of activation maps for each different filter are stacked together along the depth dimension to produce the output volume. Parameter Sharing contributes to the translation invariance of the CNN architecture.
It is important to notice that sometimes the parameter sharing assumption may not make sense. This is especially the case when the input images to a CNN have some specific centered structure, in which we expect completely different features to be learned on different spatial locations. One practical example is when the input are faces that have been centered in the image: we might expect different eye-specific or hair-specific features to be learned in different parts of the image. In that case it is common to relax the parameter sharing scheme, and instead simply call the layer a locally connected layer.
Pooling layer
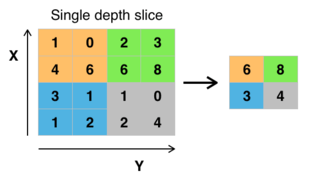
Another important concept of CNNs is pooling, which is a form of non-linear down-sampling. There are several non-linear functions to implement pooling among which max pooling is the most common. It partitions the input image into a set of non-overlapping rectangles and, for each such sub-region, outputs the maximum. The intuition is that once a feature has been found, its exact location isn't as important as its rough location relative to other features. The function of the pooling layer is to progressively reduce the spatial size of the representation to reduce the amount of parameters and computation in the network, and hence to also control overfitting. It is common to periodically insert a pooling layer in-between successive conv layers in a CNN architecture. The pooling operation provides a form of translation invariance.
The pooling layer operates independently on every depth slice of the input and resizes it spatially. The most common form is a pooling layer with filters of size 2x2 applied with a stride of 2 downsamples at every depth slice in the input by 2 along both width and height, discarding 75% of the activations. Every MAX operation would in this case be taking a max over 4 numbers. The depth dimension remains unchanged.
In addition to max pooling, the pooling units can also perform other functions, such as average pooling and even L2-norm pooling. Average pooling was often used historically but has recently fallen out of favor compared to the max pooling operation, which has been found to work better in practice.
Due to the aggressive reduction in the size of the representation (which is helpful only for smaller datasets to control overfitting), the current trend in the literature is towards using smaller filters[33] or discarding the pooling layer altogether.[34]
ReLU layer
ReLU is the abbreviation of Rectified Linear Units. This is a layer of neurons that applies the non-saturating activation function . It increases the nonlinear properties of the decision function and of the overall network without affecting the receptive fields of the convolution layer.
Other functions are also used to increase nonlinearity, for example the saturating hyperbolic tangent , , and the sigmoid function . Compared to other functions the usage of ReLU is preferable, because it results in the neural network training several times faster,[35] without making a significant difference to generalisation accuracy.
Fully connected layer
Finally, after several convolutional and max pooling layers, the high-level reasoning in the neural network is done via fully connected layers. Neurons in a fully connected layer have full connections to all activations in the previous layer, as seen in regular Neural Networks. Their activations can hence be computed with a matrix multiplication followed by a bias offset.
Loss layer
The loss layer specifies how the network training penalizes the deviation between the predicted and true labels and is normally the last layer in the network. Various loss functions appropriate for different tasks may be used there. Softmax loss is used for predicting a single class of K mutually exclusive classes. Sigmoid cross-entropy loss is used for predicting K independent probability values in . Euclidean loss is used for regressing to real-valued labels .
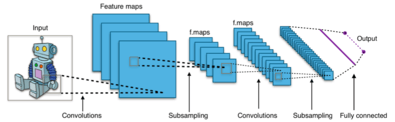
Choosing hyperparameters
CNNs use more hyperparameters than a standard MLP. While the usual rules for learning rates and regularization constants still apply, the following should be kept in mind when optimising convolutional networks.
Number of filters
Since feature map size decreases with depth, layers near the input layer will tend to have fewer filters while layers higher up can have more. To equalize computation at each layer, the product of the number of features and the number of pixel positions is typically picked to be roughly constant across layers. Preserving the information about the input would require keeping the total number of activations (number of feature maps times number of pixel positions) to be non-decreasing from one layer to the next.
The number of feature maps directly controls capacity and depends on the number of available examples and the complexity of the task.
Filter shape
Common field shapes found in the literature vary greatly, and are usually chosen based on the dataset. Best results on MNIST-sized images (28x28) are usually in the 5x5 range on the first layer, while natural image datasets (often with hundreds of pixels in each dimension) tend to use larger first-layer filters of shape 12x12 or 15x15.
The challenge is thus to find the right level of granularity so as to create abstractions at the proper scale, given a particular dataset.
Max Pooling Shape
Typical values are 2x2. Very large input volumes may warrant 4x4 pooling in the lower-layers. However, choosing larger shapes will dramatically reduce the dimension of the signal, and may result in discarding too much information.
Regularization methods
Empirical
Dropout
Since a fully connected layer occupies most of the parameters, it is prone to overfitting. The dropout method[36][37] is introduced to prevent overfitting. At each training stage, individual nodes are either "dropped out" of the net with probability or kept with probability , so that a reduced network is left; incoming and outgoing edges to a dropped-out node are also removed. Only the reduced network is trained on the data in that stage. The removed nodes are then reinserted into the network with their original weights.
In the training stages, the probability a hidden node will be retained (i.e. not dropped) is usually 0.5; for input nodes the retention probability should be much higher, intuitively because information is directly lost when input nodes are ignored.
At testing time after training has finished, we would ideally like to find a sample average of all possible dropped-out networks; unfortunately this is unfeasible for large values of . However, we can find an approximation by using the full network with each node's output weighted by a factor of , so the expected value of the output of any node is the same as in the training stages. This is the biggest contribution of the dropout method: although it effectively generates neural nets, and as such allows for model combination, at test time only a single network needs to be tested.
By avoiding training all nodes on all training data, dropout decreases overfitting in neural nets. The method also significantly improves the speed of training. This makes model combination practical, even for deep neural nets. The technique seems to reduce the complex, tightly fitted interactions between nodes, leading them to learn more robust features which better generalize to new data. Dropout has been shown to improve the performance of neural networks on tasks in vision, speech recognition, document classification, and computational biology.
DropConnect
DropConnect[38] is the generalization of Dropout in which each connection, rather than each output unit, can be dropped with probability . Each unit thus receives input from a random subset of units in the previous layer.
DropConnect is similar to Dropout as it introduces dynamic sparsity within the model, but differs in that the sparsity is on the weights, rather than the output vectors of a layer. In other words, the fully connected layer with DropConnect becomes a sparsely connected layer in which the connections are chosen at random during the training stage.
Stochastic pooling
A major drawback to Dropout is that it does not have the same benefits for convolutional layers, where the neurons are not fully connected.
In stochastic pooling,[39] the conventional deterministic pooling operations are replaced with a stochastic procedure, where the activation within each pooling region is picked randomly according to a multinomial distribution, given by the activities within the pooling region. The approach is hyper-parameter free and can be combined with other regularization approaches, such as dropout and data augmentation.
An alternate view of stochastic pooling is that it is equivalent to standard max pooling but with many copies of an input image, each having small local deformations. This is similar to explicit elastic deformations of the input images,[40] which delivers excellent MNIST performance. Using stochastic pooling in a multilayer model gives an exponential number of deformations since the selections in higher layers are independent of those below.
Artificial data
Since the degree of overfitting of a model is determined by both its power and the amount of training it receives, providing a convolutional network with extra training examples can reduce overfitting. Since these networks are usually already trained with all available data, one approach is to either generate new examples from scratch (if possible) or perturb the existing training samples to create new ones. For example, input images could be asymmetrically cropped by a few percent to create new examples with the same label as the original.[41]
Explicit
Early stopping
One of the simplest methods to prevent overfitting of a network is to simply stop the training before overfitting has had a chance to occur. It is very easy to see why this technique works, but it comes with the disadvantage that the learning process is halted.
Number of parameters
Another very simple way to prevent overfitting of a network is to limit the number parameters in the network, typically by limiting the number of hidden units in each layer or the depth of the network. For convolutional networks, the filter size also affects the number of parameters in the network. Limiting the number of parameters restricts the predictive power of the network directly, reducing the complexity of the function that it can perform on the data, and thus limits the amount of overfitting. This is equivalent to a "zero norm".
Weight decay
A simple form of added regularizer is weight decay, which simply adds an additional error, proportional to the sum of weights (L1 norm) or squared magnitude (L2 norm) of the weight vector, to the error at each node. The level of acceptable model complexity can be reduced by increasing the proportionality constant, thus increasing the penalty for large weight vectors.
L2 regularization is perhaps the most common form of regularization. It can be implemented by penalizing the squared magnitude of all parameters directly in the objective. The L2 regularization has the intuitive interpretation of heavily penalizing peaky weight vectors and preferring diffuse weight vectors. Due to multiplicative interactions between weights and inputs this has the appealing property of encouraging the network to use all of its inputs a little rather than some of its inputs a lot.
L1 regularization is another relatively common form of regularization. It is possible to combine the L1 regularization with the L2 regularization (this is called Elastic net regularization). The L1 regularization has the intriguing property that it leads the weight vectors to become sparse during optimization. In other words, neurons with L1 regularization end up using only a sparse subset of their most important inputs and become nearly invariant to the noisy inputs.
Max norm constraints
Another form of regularization is to enforce an absolute upper bound on the magnitude of the weight vector for every neuron and use projected gradient descent to enforce the constraint. In practice, this corresponds to performing the parameter update as normal, and then enforcing the constraint by clamping the weight vector of every neuron to satisfy . Typical values of are on orders of 3 or 4. Some papers report improvements[42] when using this form of regularization.
Hierarchical coordinate frames
Pooling in convolutional networks loses the precise spatial relationships between high-level parts (such as nose and mouth in a face image). The precise spatial relationships are needed for identity recognition. Overlapping the pools so that each feature occurs in different pools, helps retain the information about the position of a feature. But convolutional nets that just use translation cannot extrapolate their understanding of geometric relationships to a radically new viewpoint, like a different orientation or a different scale. On the other hand, people are very good at extrapolating; after seeing a new shape once they can recognize it from a different viewpoint.[43]
Currently, the common way to deal with this problem is to train the convolutional nets on transformed data in different orientations, scales, lighting, etc. so that the network can cope with these variations, which is extremely computationally intensive for large data-sets. The alternative is to use a hierarchy of coordinate frames and to use a group of neurons to represent a conjunction of the shape of the feature and its pose relative to the retina. The pose relative to retina is the relationship between the coordinate frame of the retina and the intrinsic coordinate frame of the feature.[44]
Thus, one way of representing something is to embed the coordinate frame within it. Once this is done, large features can be recognized by using the consistency of the poses of their parts (e.g. nose and mouth poses make a consistent prediction of the pose of the whole face). Using this approach one can say that the higher level entity (e.g. face) is present when the lower level visual entities (e.g. nose and mouth) agree on its prediction of the pose. The vectors of neuronal activity that represent pose ("pose vectors") allow spatial transformations modeled as linear operations that make it easier for the network to learn the hierarchy of visual entities and generalize across viewpoints. This is similar to the way the human visual system imposes coordinate frames in order to represent shapes.[45]
Applications
Image recognition
Convolutional neural networks are often used in image recognition systems. They have achieved an error rate of 0.23 percent on the MNIST database, which as of February 2012 is the lowest achieved on the database.[11] Another paper on using CNN for image classification reported that the learning process was "surprisingly fast"; in the same paper, the best published results at the time were achieved in the MNIST database and the NORB database.[9]
When applied to facial recognition, they were able to contribute to a large decrease in error rate.[46] In another paper, they were able to achieve a 97.6 percent recognition rate on "5,600 still images of more than 10 subjects".[2] CNNs have been used to assess video quality in an objective way after being manually trained; the resulting system had a very low root mean square error.[12]
The ImageNet Large Scale Visual Recognition Challenge is a benchmark in object classification and detection, with millions of images and hundreds of object classes. In the ILSVRC 2014,[47] which is large-scale visual recognition challenge, almost every highly ranked team used CNN as their basic framework. The winner GoogLeNet[48] (the foundation of DeepDream) increased the mean average precision of object detection to 0.439329, and reduced classification error to 0.06656, the best result to date. Its network applied more than 30 layers. Performance of convolutional neural networks on the ImageNet tests is now close to that of humans.[49] The best algorithms still struggle with objects that are small or thin, such as a small ant on a stem of a flower or a person holding a quill in their hand. They also have trouble with images that have been distorted with filters, an increasingly common phenomenon with modern digital cameras. By contrast, those kinds of images rarely trouble humans. Humans, however, tend to have trouble with other issues. For example, they are not good at classifying objects into fine-grained categories such as the particular breed of dog or species of bird, whereas convolutional neural networks handle this with ease.
In 2015 a many-layered CNN demonstrated the ability to spot faces from a wide range of angles, including upside down, even when partially occluded with competitive performance. The network trained on a database of 200,000 images that included faces at various angles and orientations and a further 20 million images without faces. They used batches of 128 images over 50,000 iterations.[50]
Video analysis
Compared to image data domains, there is relatively little work on applying CNNs to video classification. Video is more complex than images since it has another (temporal) dimension. However, some extensions of CNNs into the video domain have been explored. One approach is to treat space and time as equivalent dimensions of the input and perform convolutions in both time and space.[51][52] Another way is to fuse the features of different convolutional neural networks, responsible for spatial and temporal stream.[53][54] Unsupervised learning schemes for training spatio-temporal features have also been introduced, based on Convolutional Gated Restricted Boltzmann Machines[55] and Independent Subspace Analysis.[56]
Natural language processing
Convolutional neural networks have also seen use in the field of natural language processing. CNN models have subsequently been shown to be effective for various NLP problems and have achieved excellent results in semantic parsing,[57] search query retrieval,[58] sentence modeling,[59] classification,[60] prediction,[61] and other traditional NLP tasks.[62]
Drug discovery
Convolutional neural networks have been used in drug discovery. Predicting the interaction between molecules and biological proteins can be used to identify potential treatments that are more likely to be effective and safe. In 2015, Atomwise introduced AtomNet, the first deep learning neural networks for structure-based rational drug design.[63] The system trains directly on 3-dimensional representations of chemical interactions. Similar to how image recognition networks learn to compose smaller, spatially proximate features into larger, complex structures,[64] AtomNet discovers chemical features, such as aromaticity, sp3 carbons, and hydrogen bonding. Subsequently, AtomNet was used to predict novel candidate biomolecules for several disease targets, most notably treatments for the Ebola virus[65] and multiple sclerosis.[66]
Playing Go
Convolutional neural networks have been used in computer Go. In December 2014, Christopher Clark and Amos Storkey published a paper showing a convolutional network trained by supervised learning from a database of human professional games could outperform GNU Go and win some games against Monte Carlo tree search Fuego 1.1 in a fraction of the time it took Fuego to play.[67] Shortly after it was announced that a large 12-layer convolutional neural network had correctly predicted the professional move in 55% of positions, equalling the accuracy of a 6 dan human player. When the trained convolutional network was used directly to play games of Go, without any search, it beat the traditional search program GNU Go in 97% of games, and matched the performance of the Monte Carlo tree search program Fuego simulating ten thousand playouts (about a million positions) per move.[68]
A couple of CNNs for choosing moves to try ("policy network") and evaluating positions ("value network") driving MCTS were used by AlphaGo, Google Deepmind's program that was the first to beat a professional human player.[69]
Fine-tuning
For many applications, only a small amount of training data is available. Convolutional neural networks usually require a large amount of training data in order to avoid overfitting. A common technique is to train the network on a larger data set from a related domain. Once the network parameters have converged an additional training step is performed using the in-domain data to fine-tune the network weights. This allows convolutional networks to be successfully applied to problems with small training sets.[70]
Common libraries
- Caffe: A popular library for convolutional neural networks. Created by the Berkeley Vision and Learning Center (BVLC). Fast. It supports both CPU and GPU. Developed in C++, and has Python and MATLAB wrappers.
- Deeplearning4j: Deep learning in Java and Scala on multi-GPU-enabled Spark. A general-purpose deep learning library for the JVM production stack running on a C++ scientific computing engine. Allows the creation of custom layers. Integrates with Hadoop and Kafka.
- deeplearning-hs: Deep learning in Haskell, supports computations with CUDA.
- MatConvNet: A convnet implementation in MATLAB.
- neon: The fastest framework for convolutional neural networks and Deep Learning with support for GPU and CPU backends. The front-end is in Python, while the fast kernels are written in custom shader assembly. Created by Nervana Systems, which was acquired by Intel.
- TensorFlow: Apache 2.0-licensed Theano-like library with support for CPU, GPU and Google's proprietary TPU,[71] mobile
- Theano: The reference deep-learning library for Python with an API largely compatible with the popular NumPy library. Allows user to write symbolic mathematical expressions, then automatically generates their derivatives, saving the user from having to code gradients or backpropagation. These symbolic expressions are automatically compiled to CUDA code for a fast, on-the-GPU implementation.
- Torch (www.torch.ch): A scientific computing framework with wide support for machine learning algorithms, written in C and lua. The main author is Ronan Collobert, and it is now used at Facebook AI Research and Twitter.
See also
- Neocognitron
- Convolution
- Deep learning
- Time delay neural network
- Vision processing unit
- Scale invariant feature transform
External links
- convnet-benchmarks — easy benchmarking of all public open-source implementations of convnets (with results)
- Beginner's Guide to Convolutional Neural Nets — A gentle tutorial on how convolutional nets work
- CS231n: Convolutional Neural Networks for Visual Recognition — Andrej Karpathy's Stanford CS class
References
- ↑ "Convolutional Neural Networks (LeNet) – DeepLearning 0.1 documentation". DeepLearning 0.1. LISA Lab. Retrieved 31 August 2013.
- 1 2 Matusugu, Masakazu; Katsuhiko Mori; Yusuke Mitari; Yuji Kaneda (2003). "Subject independent facial expression recognition with robust face detection using a convolutional neural network" (PDF). Neural Networks. 16 (5): 555–559. doi:10.1016/S0893-6080(03)00115-1. Retrieved 17 November 2013.
- 1 2 LeCun, Yann. "LeNet-5, convolutional neural networks". Retrieved 16 November 2013.
- ↑ van den Oord, Aaron; Dieleman, Sander; Schrauwen, Benjamin (2013-01-01). Burges, C. J. C.; Bottou, L.; Welling, M.; Ghahramani, Z.; Weinberger, K. Q., eds. Deep content-based music recommendation (PDF). Curran Associates, Inc. pp. 2643–2651.
- ↑ Collobert, Ronan; Weston, Jason (2008-01-01). "A Unified Architecture for Natural Language Processing: Deep Neural Networks with Multitask Learning". Proceedings of the 25th International Conference on Machine Learning. ICML '08. New York, NY, USA: ACM: 160–167. doi:10.1145/1390156.1390177. ISBN 978-1-60558-205-4.
- 1 2 Zhang, Wei (1988). "Shift-invariant pattern recognition neural network and its optical architecture". Proceedings of annual conference of the Japan Society of Applied Physics.
- 1 2 Zhang, Wei (1990). "Parallel distributed processing model with local space-invariant interconnections and its optical architecture". Applied Optics. 29 (32).
- ↑ Korekado, Keisuke; Morie, Takashi; Nomura, Osamu; Ando, Hiroshi; Nakano, Teppei; Matsugu, Masakazu; Iwata, Atsushi (2003). "A Convolutional Neural Network VLSI for Image Recognition Using Merged/Mixed Analog-Digital Architecture". Knowledge-Based Intelligent Information and Engineering Systems: 169–176. CiteSeerX 10.1.1.125.3812
.
- 1 2 3 Ciresan, Dan; Ueli Meier; Jonathan Masci; Luca M. Gambardella; Jurgen Schmidhuber (2011). "Flexible, High Performance Convolutional Neural Networks for Image Classification" (PDF). Proceedings of the Twenty-Second international joint conference on Artificial Intelligence-Volume Volume Two. 2: 1237–1242. Retrieved 17 November 2013.
- ↑ Krizhevsky, Alex. "ImageNet Classification with Deep Convolutional Neural Networks" (PDF). Retrieved 17 November 2013.
- 1 2 3 4 Ciresan, Dan; Meier, Ueli; Schmidhuber, Jürgen (June 2012). "Multi-column deep neural networks for image classification". 2012 IEEE Conference on Computer Vision and Pattern Recognition. New York, NY: Institute of Electrical and Electronics Engineers (IEEE): 3642–3649. arXiv:1202.2745v1
. doi:10.1109/CVPR.2012.6248110. ISBN 978-1-4673-1226-4. OCLC 812295155. Retrieved 2013-12-09.
- 1 2 Le Callet, Patrick; Christian Viard-Gaudin; Dominique Barba (2006). "A Convolutional Neural Network Approach for Objective Video Quality Assessment" (PDF). IEEE Transactions on Neural Networks. 17 (5): 1316–1327. doi:10.1109/TNN.2006.879766. PMID 17001990. Retrieved 17 November 2013.
- ↑ Hubel, D. H.; Wiesel, T. N. (1968-03-01). "Receptive fields and functional architecture of monkey striate cortex". The Journal of Physiology. 195 (1): 215–243. doi:10.1113/jphysiol.1968.sp008455. ISSN 0022-3751. PMC 1557912
. PMID 4966457.
- ↑ LeCun, Yann; Bengio, Yoshua; Hinton, Geoffrey (2015). "Deep learning". Nature. 521 (7553): 436–444. doi:10.1038/nature14539. PMID 26017442.
- ↑ Fukushima, Kunihiko (1980). "Neocognitron: A Self-organizing Neural Network Model for a Mechanism of Pattern Recognition Unaffected by Shift in Position" (PDF). Biological Cybernetics. 36 (4): 193–202. doi:10.1007/BF00344251. PMID 7370364. Retrieved 16 November 2013.
- ↑ David E. Rumelhart; Geoffrey E. Hinton; Ronald J. Wiliams (1986). "Chapter 8 : Learning Internal Representations by ErrorPropagation". In Rumelhart, David E.; McClelland, James.L. Parallel Distributed Processing, Volume 1 (PDF). MIT Press. pp. 319–362. ISBN 9780262680530.
- ↑ Homma, Toshiteru; Les Atlas; Robert Marks II (1988). "An Artificial Neural Network for Spatio-Temporal Bipolar Patters: Application to Phoneme Classification" (PDF). Advances in Neural Information Processing Systems. 1: 31–40.
- 1 2 LeCun, Yann; Léon Bottou; Yoshua Bengio; Patrick Haffner (1998). "Gradient-based learning applied to document recognition" (PDF). Proceedings of the IEEE. 86 (11): 2278–2324. doi:10.1109/5.726791. Retrieved October 7, 2016.
- ↑ S. Behnke. Hierarchical Neural Networks for Image Interpretation, volume 2766 of Lecture Notes in Computer Science. Springer, 2003.
- ↑ Simard, Patrice, David Steinkraus, and John C. Platt. "Best Practices for Convolutional Neural Networks Applied to Visual Document Analysis." In ICDAR, vol. 3, pp. 958-962. 2003.
- ↑ Zhang, Wei (1991). "Error Back Propagation with Minimum-Entropy Weights: A Technique for Better Generalization of 2-D Shift-Invariant NNs". Proceedings of the International Joint Conference on Neural Networks.
- ↑ Zhang, Wei (1991). "Image processing of human corneal endothelium based on a learning network". Applied Optics. 30 (29).
- ↑ Zhang, Wei (1994). "Computerized detection of clustered microcalcifications in digital mammograms using a shift-invariant artificial neural network". Medical Physics. 21 (4).
- ↑ Daniel Graupe, Ruey Wen Liu, George S Moschytz."Applications of neural networks to medical signal processing". In Proc. 27th IEEE Decision and Control Conf., pp. 343-347, 1988.
- ↑ Daniel Graupe, Boris Vern, G. Gruener, Aaron Field, and Qiu Huang. "Decomposition of surface EMG signals into single fiber action potentials by means of neural network". Proc. IEEE International Symp. on Circuits and Systems , pp. 1008–1011, 1989.
- ↑ Qiu Huang, Daniel Graupe, Yi Fang Huang, Ruey Wen Liu."Identification of firing patterns of neuronal signals." In Proc. 28th IEEE Decision and Control Conf., pp. 266-271, 1989.
- ↑ Dave Steinkraus; Patrice Simard; Ian Buck (2005). "Using GPUs for Machine Learning Algorithms". 12th International Conference on Document Analysis and Recognition (ICDAR 2005). pp. 1115–1119.
- ↑ Kumar Chellapilla; Sid Puri; Patrice Simard (2006). "High Performance Convolutional Neural Networks for Document Processing". In Lorette, Guy. Tenth International Workshop on Frontiers in Handwriting Recognition. Suvisoft.
- ↑ Hinton, GE; Osindero, S; Teh, YW (Jul 2006). "A fast learning algorithm for deep belief nets.". Neural computation. 18 (7): 1527–54. doi:10.1162/neco.2006.18.7.1527. PMID 16764513.
- ↑ Bengio, Yoshua; Lamblin, Pascal; Popovici, Dan; Larochelle, Hugo (2007). "Greedy Layer-Wise Training of Deep Networks". Advances in Neural Information Processing Systems: 153–160.
- ↑ Ranzato, MarcAurelio; Poultney, Christopher; Chopra, Sumit; LeCun, Yann (2007). "Efficient Learning of Sparse Representations with an Energy-Based Model" (PDF). Advances in Neural Information Processing Systems.
- ↑ 10. Deng, Jia, et al. "Imagenet: A large-scale hierarchical image database."Computer Vision and Pattern Recognition, 2009. CVPR 2009. IEEE Conference on. IEEE, 2009.
- ↑ Graham, Benjamin (2014-12-18). "Fractional Max-Pooling". arXiv:1412.6071
[cs.CV].
- ↑ Springenberg, Jost Tobias; Dosovitskiy, Alexey; Brox, Thomas; Riedmiller, Martin (2014-12-21). "Striving for Simplicity: The All Convolutional Net". arXiv:1412.6806
[cs.LG].
- ↑ Krizhevsky, A.; Sutskever, I.; Hinton, G. E. (2012). "Imagenet classification with deep convolutional neural networks". Advances in Neural Information Processing Systems. 1: 1097–1105.
- ↑ Srivastava, Nitish; C. Geoffrey Hinton; Alex Krizhevsky; Ilya Sutskever; Ruslan Salakhutdinov (2014). "Dropout: A Simple Way to Prevent Neural Networks from overfitting" (PDF). Journal of Machine Learning Research. 15 (1): 1929–1958.
- ↑ Carlos E. Perez. "A Pattern Language for Deep Learning".
- ↑ "Regularization of Neural Networks using DropConnect | ICML 2013 | JMLR W&CP". jmlr.org. Retrieved 2015-12-17.
- ↑ Zeiler, Matthew D.; Fergus, Rob (2013-01-15). "Stochastic Pooling for Regularization of Deep Convolutional Neural Networks". arXiv:1301.3557
[cs.LG].
- ↑ "Best Practices for Convolutional Neural Networks Applied to Visual Document Analysis – Microsoft Research". research.microsoft.com. Retrieved 2015-12-17.
- ↑ Hinton, Geoffrey E.; Srivastava, Nitish; Krizhevsky, Alex; Sutskever, Ilya; Salakhutdinov, Ruslan R. (2012). "Improving neural networks by preventing co-adaptation of feature detectors". arXiv:1207.0580
[cs.NE].
- ↑ "Dropout: A Simple Way to Prevent Neural Networks from Overfitting". jmlr.org. Retrieved 2015-12-17.
- ↑ Hinton, Geoffrey. "Some demonstrations of the effects of structural descriptions in mental imagery." Cognitive Science 3.3 (1979): 231-250.
- ↑ Rock, Irvin. "The frame of reference." The legacy of Solomon Asch: Essays in cognition and social psychology (1990): 243-268.
- ↑ J. Hinton, Coursera lectures on Neural Networks, 2012, Url: https://www.coursera.org/course/neuralnets
- ↑ Lawrence, Steve; C. Lee Giles; Ah Chung Tsoi; Andrew D. Back (1997). "Face Recognition: A Convolutional Neural Network Approach". Neural Networks, IEEE Transactions on. 8 (1): 98–113. CiteSeerX 10.1.1.92.5813
. doi:10.1109/72.554195.
- ↑ "ImageNet Large Scale Visual Recognition Competition 2014 (ILSVRC2014)". Retrieved 30 January 2016.
- ↑ Szegedy, Christian; Liu, Wei; Jia, Yangqing; Sermanet, Pierre; Reed, Scott; Anguelov, Dragomir; Erhan, Dumitru; Vanhoucke, Vincent; Rabinovich, Andrew (2014). "Going Deeper with Convolutions". Computing Research Repository. arXiv:1409.4842
.
- ↑ Russakovsky, Olga; Deng, Jia; Su, Hao; Krause, Jonathan; Satheesh, Sanjeev; Ma, Sean; Huang, Zhiheng; Karpathy, Andrej; Khosla, Aditya; Bernstein, Michael; Berg, Alexander C.; Fei-Fei, Li (2014). "Image Net Large Scale Visual Recognition Challenge". arXiv:1409.0575
[cs.CV].
- ↑ "The Face Detection Algorithm Set To Revolutionize Image Search". Technology Review. February 16, 2015. Retrieved February 2015. Check date values in:
|access-date=
(help) - ↑ Baccouche, Moez; Mamalet, Franck; Wolf, Christian; Garcia, Christophe; Baskurt, Atilla (2011-11-16). "Sequential Deep Learning for Human Action Recognition". In Salah, Albert Ali; Lepri, Bruno. Human Behavior Unterstanding. Lecture Notes in Computer Science. 7065. Springer Berlin Heidelberg. pp. 29–39. doi:10.1007/978-3-642-25446-8_4. ISBN 978-3-642-25445-1.
- ↑ Ji, Shuiwang; Xu, Wei; Yang, Ming; Yu, Kai (2013-01-01). "3D Convolutional Neural Networks for Human Action Recognition". IEEE Transactions on Pattern Analysis and Machine Intelligence. 35 (1): 221–231. doi:10.1109/TPAMI.2012.59. ISSN 0162-8828. PMID 22392705.
- ↑ Karpathy, Andrej, et al. "Large-scale video classification with convolutional neural networks." IEEE Conference on Computer Vision and Pattern Recognition (CVPR). 2014.
- ↑ Simonyan, Karen; Zisserman, Andrew (2014). "Two-Stream Convolutional Networks for Action Recognition in Videos". arXiv:1406.2199
[cs.CV]. (2014).
- ↑ Taylor, Graham W.; Fergus, Rob; LeCun, Yann; Bregler, Christoph (2010-01-01). "Convolutional Learning of Spatio-temporal Features". Proceedings of the 11th European Conference on Computer Vision: Part VI. ECCV'10. Berlin, Heidelberg: Springer-Verlag: 140–153. ISBN 3-642-15566-9.
- ↑ Le, Q. V.; Zou, W. Y.; Yeung, S. Y.; Ng, A. Y. (2011-01-01). "Learning Hierarchical Invariant Spatio-temporal Features for Action Recognition with Independent Subspace Analysis". Proceedings of the 2011 IEEE Conference on Computer Vision and Pattern Recognition. CVPR '11. Washington, DC, USA: IEEE Computer Society: 3361–3368. doi:10.1109/CVPR.2011.5995496. ISBN 978-1-4577-0394-2.
- ↑ Grefenstette, Edward; Blunsom, Phil; de Freitas, Nando; Hermann, Karl Moritz (2014-04-29). "A Deep Architecture for Semantic Parsing". arXiv:1404.7296
[cs.CL].
- ↑ "Learning Semantic Representations Using Convolutional Neural Networks for Web Search – Microsoft Research". research.microsoft.com. Retrieved 2015-12-17.
- ↑ Kalchbrenner, Nal; Grefenstette, Edward; Blunsom, Phil (2014-04-08). "A Convolutional Neural Network for Modelling Sentences". arXiv:1404.2188
[cs.CL].
- ↑ Kim, Yoon (2014-08-25). "Convolutional Neural Networks for Sentence Classification". arXiv:1408.5882
[cs.CL].
- ↑ Collobert, Ronan, and Jason Weston. "A unified architecture for natural language processing: Deep neural networks with multitask learning."Proceedings of the 25th international conference on Machine learning. ACM, 2008.
- ↑ Collobert, Ronan; Weston, Jason; Bottou, Leon; Karlen, Michael; Kavukcuoglu, Koray; Kuksa, Pavel (2011-03-02). "Natural Language Processing (almost) from Scratch". arXiv:1103.0398
[cs.LG].
- ↑ Wallach, Izhar; Dzamba, Michael; Heifets, Abraham (2015-10-09). "AtomNet: A Deep Convolutional Neural Network for Bioactivity Prediction in Structure-based Drug Discovery". arXiv:1510.02855
[cs.LG].
- ↑ Yosinski, Jason; Clune, Jeff; Nguyen, Anh; Fuchs, Thomas; Lipson, Hod (2015-06-22). "Understanding Neural Networks Through Deep Visualization". arXiv:1506.06579
[cs.CV].
- ↑ "Toronto startup has a faster way to discover effective medicines". The Globe and Mail. Retrieved 2015-11-09.
- ↑ "Startup Harnesses Supercomputers to Seek Cures". KQED Future of You. Retrieved 2015-11-09.
- ↑ Clark, Christopher; Storkey, Amos (2014). "Teaching Deep Convolutional Neural Networks to Play Go". arXiv:1412.3409
[cs.AI].
- ↑ Maddison, Chris J.; Huang, Aja; Sutskever, Ilya; Silver, David (2014). "Move Evaluation in Go Using Deep Convolutional Neural Networks". arXiv:1412.6564
[cs.LG].
- ↑ "AlphaGo – Google DeepMind". Retrieved 30 January 2016.
- ↑ Durjoy Sen Maitra; Ujjwal Bhattacharya; S.K. Parui, , "CNN based common approach to handwritten character recognition of multiple scripts," in Document Analysis and Recognition (ICDAR), 2015 13th International Conference on , vol., no., pp.1021-1025, 23-26 Aug. 2015
- ↑ Cade Metz (May 18, 2016). "Google Built Its Very Own Chips to Power Its AI Bots". Wired.